No products in the cart.
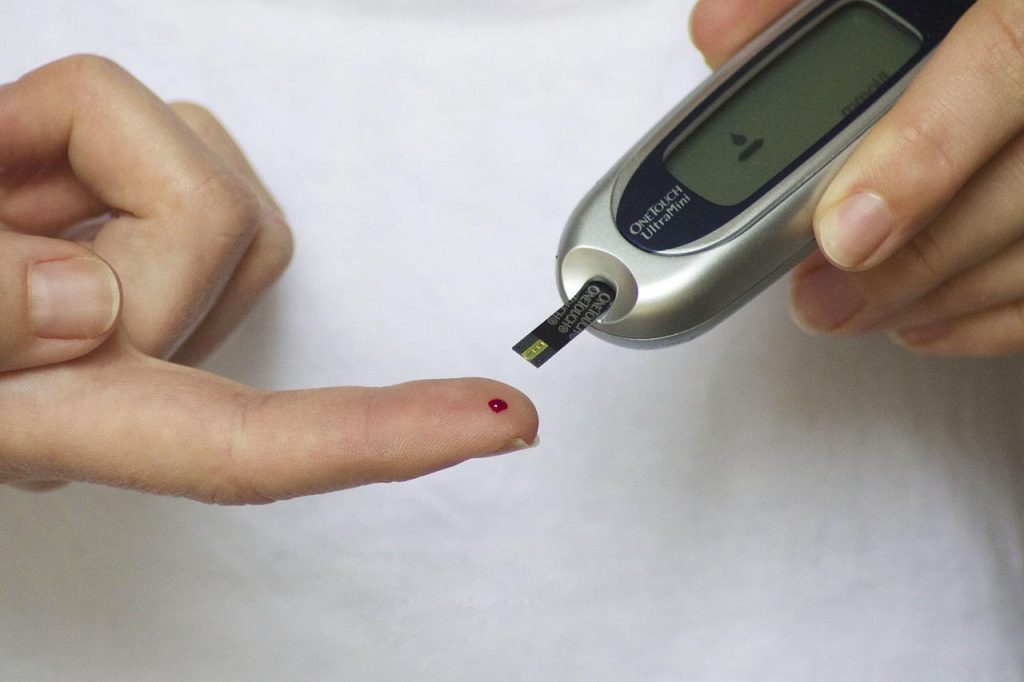
Dimethylglycine Deficiency and the Development of Diabetes
GENETICS / GENOMES / PROTEOMICS / METABOLOMICS
Martin Magnusson; Thomas J. Wang; Clary Clish; Gunnar Engström; Peter Nilsson; Robert E. Gerszten; Olle Melander
Corresponding author: Martin Magnusson, martin.magnusson@med.lu.se.Diabetes 2015;64(8):3010–3016https://doi.org/10.2337/db14-1863Article history
Experimental studies have suggested possible protective effects of dimethylglycine (DMG) on glucose metabolism. DMG is degraded to glycine through a DMG-dehydrogenase (DMGDH)-catalyzed reaction, and this is the only known pathway for the breakdown of DMG in mammals. In this study, we aimed to identify the strongest genetic determinant of circulating DMG concentration and to investigate its associations with metabolic traits and incident diabetes. In the cohort with full metabolomics data (n = 709), low plasma levels of DMG were significantly associated with higher blood glucose levels (P = 3.9E–4). In the genome-wide association study (GWAS) of the discovery cohort (n = 5,205), the strongest genetic signal of plasma DMG was conferred by rs2431332 at the DMGDH locus, where the major allele was associated with lower DMG levels (P = 2.5E–15). The same genetic variant (major allele of rs2431332) was also significantly associated with higher plasma insulin (P = 0.019), increased HOMA insulin resistance (P = 0.019), and an increased risk of incident diabetes (P = 0.001) in the pooled analysis of the discovery cohort together with the two replication cohorts (n = 20,698 and n = 7,995). These data are consistent with a possible causal role of DMG deficiency in diabetes development and encourage future studies examining if inhibition of DMGDH, or alternatively, supplementation of DMG, might prove useful for the treatment/prevention of diabetes.
Introduction
Using mass spectrometry (MS)-based metabolomic approaches, recent studies have identified associations between small molecules and insulin sensitivity and type 2 diabetes (1–4). Although these circulating metabolites may represent useful markers of disease susceptibility, their causal involvement in the development of diabetes is less certain. For example, although previous studies have shown that high glycine levels are associated with increased insulin sensitivity and decreased type 2 diabetes risk (5–7), a recent study failed to show association between a genetic variant within the CPS-1 gene that was genome-wide significantly associated with glycine, with increased insulin sensitivity and decreased risk of diabetes arguing against a causal involvement of glycine in diabetes development (8).
The clinical importance of assessing causality between circulating biomarkers and disease with the use of Mendelian randomization approaches has been clearly exemplified by genetic studies of LDL- and HDL-cholesterol (9). This proof-of-concept study showed that whereas genetic elevation of LDL-cholesterol associated with coronary artery disease (CAD), genetically lower levels of HDL-cholesterol did not, results that are in concert with the CAD preventive effect of statins and the lack of such effect of drugs elevating HDL-cholesterol by inhibition of cholesteryl ester transfer protein (10,11).
The tertiary amine dimethylglycine (DMG) is produced from betaine during the remethylation of homocysteine to methionine, catalyzed by betaine-homo-cysteine methyltransferase (BHMT). DMG is in turn catalyzed by DMG dehydrogenase (DMGDH) and sarcosine dehydrogenase metabolized to glycine. Epidemiological data have shown that plasma levels of betaine are associated in opposite directions with key components of the metabolic syndrome (12), and high plasma levels of glycine have been suggested to be associated with increased insulin sensitivity. However, data on their intermediate metabolite DMG in relation to diabetes development is lacking.
The first aim of this study was to examine the association of plasma levels of DMG and glycine with glycemia and insulin resistance. Secondly, we aimed to replicate genetic associations with DMG (DMGDH locus) (13) and glycine (CPS-1) (8,14) and to investigate whether genetic alterations underlying DMG and glycine concentrations are associated with glucometabolic risk factors and incident diabetes.
Research Design and Methods
Study Samples
Metabolite Cohort
Metabolites were profiled from EDTA plasma collected at the baseline examination of participants of the Malmö Diet and Cancer Study–Cardiovascular Cohort (MDC-CC; see below for a description of the MDC-CC) using previously described methodology (3,15) (see also the section 9metabolic profiling for details). These subjects were derived from a nested incident cardiovascular disease (CVD) case-control study (n = 506) (16) with case and control subjects matched by sex, age, and Framingham risk score (17), and a nested incident diabetes case-control study (n = 326) (3). From this pool, 27 subjects who participated in both studies were excluded, leaving 805 subjects. Of these, 709 were successfully genotyped for the genome-wide association study (GWAS) (see genotyping), passed all quality control steps, and had complete data on all covariates (age, sex, BMI). These 709 subjects in the metabolite cohort were used in a GWAS analysis to identify genetic variants associated with the levels of DMG and glycine in plasma. In addition, the metabolite cohort was used to study the relationship between plasma levels of DMG and glycine in relation to metabolic traits (glucose, insulin, and HOMA of insulin resistance [HOMA-index]).
The MDC
The MDC is a prospective population-based study (n = 30,447) where baseline examinations, including anthropometric measurements and blood sample donations including DNA, were performed between 1991 and 1996 (18). A complete description of the study population has been given elsewhere (19).
The MDC-CC
To study the epidemiology of carotid artery disease, a random sample of the MDC study subjects was selected (n = 6,103) during the years 1991–1994, and this sample is referred to as the MDC-CC (20,21). Fasting plasma samples were obtained in 5,405 subjects in the MDC-CC (20). In MDC-CC subjects for whom GWAS genotyping was performed and data were available on fasting glucose and insulin, cross-sectional analyses were performed between plasma DMG and glycine-associated single nucleotide polymorphisms (SNPs) (rs2431332 at the DMGDH locus and rs4673546 at the CPS-1 locus) and plasma glucose (n = 4,790), insulin (n = 4,740), and HOMA-index (n = 4,745). Furthermore, after exclusion of subjects in the MDC-CC with prevalent diabetes at the baseline examination (n = 242), we related rs2431332 and rs4673546 to the incidence of diabetes in 5,205 subjects during a median (interquartile range [IQR]) follow-up time of 16.6 (14.5–18.7) years using local and national registries as described below.
The MDC Replication Cohort
After excluding 1,044 subjects with prevalent diabetes at the baseline examination among MDC participants who were not included in the MDC-CC, we genotyped an additional 20,698 MDC subjects (the MDC replication cohort) for the rs2431332 and rs4673546 using the TaqMan system (see genotyping) for the analysis of these genetic variants and incidence of diabetes during a median (IQR) follow-up time of 14.6 (12.1–17.0) years (see clinical assessment).
The Malmö Preventive Project
The Malmö Preventive Project (MPP, 1974–1992) was a preventive program with the aim to screen for cardiovascular risk factors, alcohol abuse, and breast cancer among inhabitants in Malmö, Sweden, born 1921–1949 (n = 33,346). A complete description of the study population has been given elsewhere (MPP baseline examination) (22). A reexamination was conducted during 2002–2006 (n = 18,240; 72% attendance rate, 63% men, mean age 69 ± 6 years) at which whole-blood DNA samples were collected. After excluding subjects who had also participated in the MDC and subjects with prevalent diabetes at the MPP baseline examination, we genotyped the rs2431332 and rs4673546 variants in 7,995 unique subjects of the MPP using the TaqMan system (see genotyping) and related the genetic variants to incidence of diabetes (see clinical assessment) during a median (IQR) follow-up time of 28.8 (25.4–30.4) years after the MPP baseline examination.
The study protocols were approved by the Lund University Institutional Review Boards, Sweden. All study participants provided written informed consent.
Metabolic Profiling
Given the relatively high analytical demands and costs of liquid chromatography–MS (LC-MS) profiling, we were not able to analyze the entire MDC-CC cohort (3,16). Plasma metabolites were profiled in EDTA plasma samples drawn at the baseline examination in the MDC-CC. Samples were stored at −80°C and profiled using LC-MS as described in detail previously (3). Formic acid, ammonium acetate, LC-MS grade solvents, and valine-d8 were purchased from Sigma-Aldrich (St. Louis, MO). The remainder of the isotopically labeled analytical standards were purchased from Cambridge Isotope Laboratories, Inc. (Andover, MA). Plasma samples (10 μL) were prepared for LC-MS analyses by protein precipitation with the addition of nine volumes of 74.9:24.9:0.2 v/v/v acetonitrile/methanol/formic acid containing two additional stable isotope-labeled internal standards for valine-d8 and phenylalanine-d8. The samples were centrifuged for 10 min at 10,000 rpm and 4°C, and the supernatants were injected directly. For all isotope standards used, peak areas were two or more orders of magnitude above the lower limit of quantification (as defined as a discrete peak 10-fold greater than noise) and fell well within the linear range of the dose-response relationship.
LC-MS data were acquired using a 4000 QTRAP triple quadrupole mass spectrometer (Applied Biosystems/SCIEX; Foster City, CA) that was coupled to a multiplexed LC system with two 1200 Series pumps (Agilent Technologies, Santa Clara, CA) and an HTS PAL autosampler (Leap Technologies, Carrboro, NC) equipped with two injection ports and a column selection valve. The two pumps were similarly configured for hydrophilic interaction chromatography using 150- × 2.1-mm Atlantis hydrophilic interaction chromatography columns (Waters, Milford, MA) and with the same mobile phases (mobile phase A: 10 mmol/L ammonium formate and 0.1% formic acid, v/v; mobile phase B: acetonitrile with 0.1% formic acid, v/v). Multiplexing was used to enable the measurement of 61 metabolite transitions divided between the 2 LC systems, and each sample was injected once on each. Each column was eluted isocratically with 5% mobile phase A for 1 min, followed by a linear gradient to 60% mobile phase A over 10 min. MS analyses were carried out using electrospray ionization and multiple reaction monitoring scans in the positive ion mode. Declustering potentials and collision energies were optimized for each metabolite by infusion of reference standards before sample analyses. The dwell time for each transition was 30 ms, the ion spray voltage was 4.5 kV, and the source temperature was 425°C. Internal standard peak areas were monitored for quality control, and individual samples with peak areas differing from the group mean by more than two standard deviations were reanalyzed. MultiQuant 1.1 software (Applied Biosystems/SCIEX) was used for automated peak integration, and metabolite peaks were manually reviewed for quality of integration and compared against a known standard to confirm identity.
Clinical Assessment
MDC and MPP participants underwent similar standardized medical histories with physical examinations and laboratory assessments. Blood pressure was obtained after 10 min of rest while supine. We calculated the BMI as weight in kilograms divided by the square of the height in meters. Hypertension was defined as systolic blood pressure ≥140 mmHg or diastolic blood pressure ≥90 mmHg, or use of antihypertensive therapy. The MDC-CC fasting blood samples were analyzed for fasting blood glucose and fasting insulin according to standard procedures at the University Hospital Malmö Department of Clinical Chemistry. We used the HOMA-index (23).
Classification of Prevalent and Incident Diabetes in MDC and MPP
Prevalent diabetes at baseline in the MDC and MPP was defined as a self-reported physician diagnosis of diabetes, use of diabetes medication, a diagnosis of diabetes in any of the local and national diabetes registries before the baseline examination (see below), or fasting whole blood glucose of ≥6.1 mmol/L, when available.
Incident diabetes (occurring after the MDC and MPP baseline examinations) was retrieved through record linkage of the Swedish personal identification number with national and regional registers as follows: the Malmö HbA1c register that analyzed all HbA1c samples at the Department of Clinical Chemistry obtained in institutional and noninstitutional care in Malmö are from 1988 and onwards (24), the Swedish National Diabetes Register (25), The Regional Diabetes 2000 register of the Scania region (26), the Swedish National Patient Register covering all somatic and psychiatric hospital discharges and hospital-based outpatient care (27), Swedish Cause of Death register (28), and Swedish Prescribed Drug Register (prescription of antidiabetes medication) (29). In addition, in the parts of MDC (30) and MPP (31) that were reexamined after the two baseline examinations in the two studies, incident diabetes was also based on a measured fasting plasma glucose value ≥7.0 mmol/L or a plasma glucose value ≥11.1 mmol/L after an oral glucose tolerance test.
Genotyping
GWAS genotyping was performed using the HumanOmniExpressExome BeadChip and iScan System (Illumina, San Diego, CA) in the MDC-CC. All other genotyping (of rs2431332 and rs4673546) was performed using “assay by design” TaqMan probes and a real-time PCR assay on an ABI 7900HT (Applied Biosystems), according to the manufacturer’s instructions. Twenty percent of the samples were run in duplicates with >99.5% concordance. All genotypes were called by two different investigators. None of the SNPs (rs2431332 and rs4673546) showed any significant deviation from Hardy-Weinberg equilibrium (P > 0.05).
Statistics
Plasma levels of glycine, DMG, glucose, insulin, and HOMA-index were transformed to their natural logarithm (ln) (due to skewed distribution) and then standardized to multiples of 1 SD. Linear regression analyses adjusted for age, sex, and BMI were performed cross-sectionally between metabolite levels (DMG and glycine) and glucometabolic traits (glucose, insulin, and HOMA-index) in the metabolite cohort. A GWAS was then performed for plasma levels of DMG and glycine in the metabolite cohort using additive genetic models of inheritance and linear regression adjusting for age, sex, and BMI.
Each of the two top genetic signals for DMG and glycine were tested for an association with fasting glucose, insulin, and HOMA-index in the MDC-CC as dependent variables using linear regression with the genotype as the explanatory variable adjusted for age, sex, and BMI.
Finally, we used logistic regression analysis to test the association between the strongest genetic signals of plasma DMG (rs2431332) and glycine (rs4673546) in the GWAS and the incidence of diabetes during follow-up in a model adjusted for age, sex, and BMI in three unique sets of individuals free from diabetes at the MDC and MPP baseline examinations, the MDC-CC, the MDC replication cohort, and the MPP. The GWAS was performed using PLINK software. All other analyses were performed in SPSS 20.0 software (IBM Corp., Armonk, NY). A two-tailed P value of <0.05 was considered statistically significant.
Results
Characteristics of the study participants in the metabolite cohort, as well as the MDC-CC, MDC replication, and MPP cohorts, are reported in Table 1 and Supplementary Table 1.Table 1
Clinical characteristics of the metabolite cohort (n = 709)
Age (years) | 59.2 ± 5.6 |
Sex (% female) | 51.5 |
Current smoker (%) | 30.1 |
BMI (kg/m2) | 27.0 ± 4.6 |
Systolic blood pressure (mmHg) | 147.5 ± 18.7 |
Diastolic blood pressure (mmHg) | 89.9 ± 9.4 |
High blood pressure medication (%) | 23.3 |
Hypertension (%) | 66.7 |
Fasting glucose (mmol/L) | 5.1 (0.7) |
Fasting insulin (μU/mL) | 8.0 (6.0) |
Fasting HOMA-index | 1.7 (1.3) |
Diabetes (%) | 4.7 |
Values are displayed as mean ± SD, medians (IQR), or frequency in percent.
DMG and Glycine Plasma Levels and Association With Metabolic Traits
Lower plasma levels of DMG were significantly associated with higher glucose levels but neither with insulin levels nor the HOMA-index. Low plasma levels of glycine were significantly associated increased glucose, insulin, and HOMA-index (Table 2).Table 2
Plasma levels of glycine and DMG and correlation to metabolic traits in the MDC-CC
Metabolic trait | Standardized β-coefficient (SE) | P value | |
---|---|---|---|
DMG | Glucose | 0.122 (0.034) | <0.001 |
Insulin | 0.006 (0.034) | 0.872 | |
HOMA-index | 0.030 (0.034) | 0.377 | |
Glycine | Glucose | 0.143 (0.036) | <0.001 |
Insulin | 0.194 (0.035) | <0.001 | |
HOMA-index | 0.205 (0.035) | <0.001 |
The β-values reflect each 1-SD decrease of plasma DMG and glycine in relation to insulin, glucose, and HOMA-index expressed on a standardized scale. Regressions are adjusted for age, sex, and BMI.
Identification of the Strongest DMG- and Glycine-Associated Variance in the Genome
We identified a genome-wide significant association between the SNP rs2431332 (major allele associated with lower plasma levels of DMG) at the DMGDH locus and its enzyme substrate DMG (P = 2.5E−15), representing the strongest signal of the GWAS (Table 3). Furthermore, we identified the strongest signal of the GWAS for plasma glycine at a region near the CPS-1 gene, rs4673546, the major allele associated with higher plasma levels of glycine (P = 1.0E−12) (Table 3).Table 3
The strongest genetic signals of plasma DMG and glycine in a GWAS of participants in the MDC-CC
Locus | Trait | SNP/exon | Ch | Position | Major/minor allele | MAF | β* | SE | P value | Gene function |
---|---|---|---|---|---|---|---|---|---|---|
DMGDH | DMG | rs2431332 | 5 | 78321109 | A/G | 0.2507 | −0.467 | 0.058 | 2.5E−15 | DMGDH catalyzes oxidative demethylation of DMG to sarcosine |
CPS-1 | Glycine | rs4673546 | 2 | 211565692 | C/T | 0.1824 | −0.453 | 0.062 | 1.0E−12 | CPS-1 catalyzes the conversion of ammonia and bicarbonate to carbamyl phosphate |
Ch, chromosome; MAF, minor allele frequency.
*The β values per allele (major allele coded) in relation to metabolite levels expressed on a standardized scale. Regressions are adjusted for age, sex, and BMI.
Effects of Metabolite-Associated SNPs on Metabolic Traits
In linear regression analysis adjusted for age, sex, and BMI, the major allele of rs2431332 (associated with lower DMG levels) showed significant direct relationships with insulin (β = 0.047, P = 0.019) and the HOMA-index (β = 0.046, P = 0.019) and a borderline significant association with glucose (β = 0.040, P = 0.067). The SNP in the CPS-1 locus (major allele of rs4673546) showed no significant associations with the measured glucometabolic traits (glucose, P = 0.872; insulin, P = 0.690; and HOMA-index, P = 0.986) (Table 4).Table 4
rs2431332 and rs4673546 correlations to metabolic traits in the MDC-CC
Glucometabolic trait | Standardized β-coefficient (SE) | P value | |
---|---|---|---|
rs2431332 | |||
Glucose | 0.040 (0.022) | 0.067 | |
Insulin | 0.047 (0.020) | 0.019 | |
HOMA-index | 0.046 (0.020) | 0.019 | |
rs4673546 | |||
Glucose | −0.004 (0.024) | 0.883 | |
Insulin | 0.009 (0.022) | 0.690 | |
HOMA-index | 0.000 (0.022) | 0.986 |
The β values per allele (major allele coded) in relation to insulin, glucose, and HOMA-index expressed on a standardized scale. Regressions are adjusted for age, sex, and BMI.
DMG Deficiency and Association With Diabetes Development
During follow-up of the MDC-CC (n = 5,205), there were 711 cases of incident diabetes. The logistic regression analyses, adjusted for age, sex, and BMI, showed that the major allele of rs2431332 was significantly associated with an increased risk of diabetes (odds ratio 1.149 [95% CI 1.002–1.315], P = 0.048) (Table 5). In contrast, the major allele of rs4673546 showed no significant association with diabetes development (0.988 [0.854–1.144], P = 0.875). We then attempted to replicate the association between the major allele of rs2431332 and incident diabetes in two large, independent populations. In the MDC replication cohort (n = 20,698), there were 2,180 cases of incident diabetes during follow-up and a borderline significant association between the major allele of rs24313332 and incident diabetes (1.078 [0.998–1.164], P = 0.057) (Table 5). In the MPP (n = 7,995), there were 1,310 cases of incident diabetes during follow-up, and the major allele of the rs2431332 was significantly associated with diabetes incidence (1.116 [1.006–1.238], P = 0.038). Pooled analysis across all three cohorts (MDC-CC, MDC replication, and MPP) was significantly associated with incident diabetes (1.101 [1.041–1.165], P = 0.001) (Fig. 1).Table 5
rs2431332 and correlation to incident diabetes in three independent Swedish cohorts
rs2431332 | Odds ratio | 95% CI | P value |
---|---|---|---|
MDC-CC | 1.149 | 1.002–1.317 | 0.048 |
MDC | 1.078 | 0.998–1.164 | 0.057 |
MPP | 1.116 | 1.006–1.238 | 0.038 |
Meta-analysis | 1.101 | 1.041–1.165 | 0.001 |
Odds ratio per allele (major allele coded). Regressions are adjusted for age, sex, and BMI.Figure 1View largeDownload slide
rs2431332 and correlation to incident diabetes in three independent Swedish cohorts. OR, odds ratio. The solid squares denote the mean difference, the horizontal lines represent the 95% CIs, and the vertical line indicates no effect. The diamond denotes the weighted mean differences, with the lateral tips indicating the associated 95% CIs.
Discussion
Most recently a GWAS identified a novel genetic variant at the DMGDH loci to be strongly (P = 6.6E–33) associated with the levels of DMG in plasma (13). Nonetheless, due to the exploratory nature of the study (GWAS of 217 plasma metabolites), possible correlations of metabolite-associated genetic variants with metabolic traits were not accounted for in the study (13). We were able to confirm this previously described genome-wide significant association between variants at DMGDH and its enzyme substrate DMG (13). Interestingly, DMGDH deficiency has been described in a male patient of African descent (A-to-G transition at nucleotide 326 of the precursor coding region of the DMGDH cDNA, resulting in a histidine-to-arginine substitution at codon 109 or residue 81 of the predicted mature protein; H811R). The patient was homozygous for this mutation and had a lifelong problem with a fishlike body odor. The intensity of the odor increased with physiologic stress, such as illness, as well as during times of increased physical activity. He experienced chronic muscular fatigue beginning in adolescence, accompanied by elevated levels of the muscle form of creatine kinase in serum. However, no details regarding the metabolic status of this patient were noted (32,33).
The literature regarding the effect of DMG on glucose metabolism in humans is scarce. A recent metabolic profiling study of 377 Framingham Offspring Study participants without diabetes, in which the effect of an oral glucose challenge on plasma levels of 110 metabolites was tested, showed that plasma levels of DMG significantly decreased during an oral glucose challenge (34). Interestingly, an animal study has demonstrated that the insulin-, glucose-, and triglyceride-lowering effect by the peroxisome proliferator–activated receptor α (PPAR-α) agonist WY14 643 is accompanied with a significant reduction in DMGDH function, together with a substantial increase in the DMG concentration in urine of treated rats compared with control rats, suggesting an extension of the role of PPAR-α beyond the control of fatty acid and glucose in rodents (35).
We could also confirm previous results showing a genome-wide significant association between plasma glycine levels and a genetic variant near the CPS-1 locus (8,14). In one of these studies, plasma levels of glycine were strongly associated with fasting insulin and clamp-based measures of insulin sensitivity, although no associations were seen for the glycine-associated SNP and measurements of insulin resistance (8). These findings are concordant with our findings in which glycine plasma levels were significantly associated with glucose and measurements of insulin resistance but the glycine-associated SNP at the CPS-1 locus was not. These findings suggest that lower glycine levels might be secondary to DMG metabolism and/or to the diabetes disease process itself.
Apart from being the end product of DMG catalyzed by DMGDH and sarcosine dehydrogenase, glycine, on one hand, is also synthesized from serine, threonine, and hydroxyproline via interorgan metabolism involving primarily the liver and kidneys (36), which makes the interpretation of the SNP/metabolite associations more complex. DMG, on the other hand, is formed from the degradation of the BHMT-catalyzed reaction, and in turn, catalyzed by DMGDH and degraded to glycine; this is the only known pathway to this metabolite in mammals. Also, because DMG is synthesized from betaine during the remethylation of homocysteine to methionine, catalyzed by BHMT, it is rational that epidemiological studies have indicated metabolic protective proprieties of plasma betaine and choline (the precursor of betaine) (12). Our finding that genetic variation (major allele of rs2431332) within the DMGDH locus was associated with lower DMG concentrations, which in turn was associated with higher blood glucose and was also associated with increased insulin resistance as well as with increased risk of incident diabetes in multiple independent large cohorts, urges for further studies examining if a possible inhibition of DMHDH or supplementation of DMG might prove useful for the treatment/prevention of diabetes.
An important strength of the current study is the use of three independent well-characterized prospective cohorts that have been monitored longitudinally for diabetes incidence for decades using nationwide registers with 100% coverage and high proven accuracy (18–21). A weakness of the study is the lack of measurements of sarcosine, the intermediate product between DMG and glycine. Furthermore, our samples consisted predominantly of individuals of European ancestry, and therefore, the results of this study may not be generalizable to other racial/ethnic groups.
Given the genetic correlation between rs2431332 and measures of insulin resistance, it was surprising that plasma DMG did not correlate with insulin resistance indexes. One reason may be insufficient power. Another reason may be confounding influences on the relationship between environmentally determined DMG, which explains the major part of DMG variance, and insulin resistance compared with the unconfounded relationship between genetically determined DMG (rs2431332) and insulin resistance.
Finally, we do not have the data to elucidate the molecular consequences of rs2431332; however, because it is not in the coding region of DMGDH, it most likely increases gene expression and thus total activity.
Our results offer an indication for a potential causal role of DMG in the etiology of diabetes. Further studies are needed to investigate the mechanisms underlying this association and possibilities for preventive interventions.
Article Information
Funding. M.M. and O.M. were supported by grants from the Swedish Medical Research Council, the Swedish Heart and Lung Foundation, the Medical Faculty of Lund University, Skåne University Hospital, the Albert Påhlsson Research Foundation, the Crafoord Foundation, the Ernhold Lundströms Research Foundation, the Region Skåne, the Hulda and Conrad Mossfelt Foundation, the Southwest Skånes Diabetes Foundation, the King Gustaf V and Queen Victoria’s Foundation, the Lennart Hanssons Memorial Fund, Knut and Alice Wallenberg Foundation, and the Marianne and Marcus Wallenberg Foundation. P.N. was supported by grants from the Swedish Medical Research Council, the Swedish Heart and Lung Foundation, the Medical Faculty of Lund University, Skåne University Hospital, and the Ernhold Lundströms Research Foundation. T.J.W. and R.E.G. were supported by National Institutes of Health NO1-HC-25195 and R01-DK-HL081572, the Leducq Foundation, and the American Heart Association.
The funding organizations had no role in the design and conduct of the study; the collection, management, analysis, and interpretation of the data; or the preparation or approval of the manuscript.
Duality of Interest. No potential conflicts of interest relevant to this article were reported.
Author Contributions. M.M., T.J.W., R.E.G., and O.M. contributed to study concept and design. M.M., T.J.W., G.E., P.N., R.E.G., and O.M. acquired data. M.M., T.J.W., C.C., G.E., P.N., R.E.G., and O.M. analyzed and interpreted data. M.M., T.J.W., R.E.G., and O.M. drafted the manuscript. M.M., T.J.W., C.C., G.E., P.N., and O.M. critically revised the manuscript for important intellectual content. M.M., T.J.W., R.E.G., and O.M. contributed to statistical analysis. M.M., T.J.W., P.N., R.E.G., and O.M. obtained funding. C.C., P.N., R.E.G., and O.M. provided administrative, technical, or material support. M.M., G.E., P.N., and O.M. supervised the study. M.M. and O.M. are the guarantors of this work and, as such, had full access to all the data in the study and take responsibility for the integrity of the data and the accuracy of the data analysis.
References
1.Newgard CB, An J, Bain JR, et al. A branched-chain amino acid-related metabolic signature that differentiates obese and lean humans and contributes to insulin resistance. Cell Metab 2009;9:311–326[PubMed]Google ScholarCrossref 2.Newsholme P, Bender K, Kiely A, Brennan L. Amino acid metabolism, insulin secretion and diabetes. Biochem Soc Trans 2007;35:1180–1186[PubMed]Google ScholarCrossref 3.Wang TJ, Larson MG, Vasan RS, et al. Metabolite profiles and the risk of developing diabetes. Nat Med 2011;17:448–453[PubMed]Google ScholarCrossref 4.Wang TJ, Ngo D, Psychogios N, et al. 2-Aminoadipic acid is a biomarker for diabetes risk. J Clin Invest 2013;123:4309–4317[PubMed]Google ScholarCrossref 5.Floegel A, Stefan N, Yu Z, et al. Identification of serum metabolites associated with risk of type 2 diabetes using a targeted metabolomic approach. Diabetes 2013;62:639–648[PubMed]Google ScholarCrossref 6.Wang-Sattler R, Yu Z, Herder C, et al. Novel biomarkers for pre-diabetes identified by metabolomics. Mol Syst Biol 2012;8:615[PubMed]Google ScholarCrossref 7.Michaliszyn SF, Sjaarda LA, Mihalik SJ, et al. Metabolomic profiling of amino acids and β-cell function relative to insulin sensitivity in youth. J Clin Endocrinol Metab 2012;97:E2119–E2124[PubMed]Google ScholarCrossref 8.Xie W, Wood AR, Lyssenko V, et al.; MAGIC Investigators; DIAGRAM Consortium; GENESIS Consortium; RISC Consortium. Genetic variants associated with glycine metabolism and their role in insulin sensitivity and type 2 diabetes. Diabetes 2013;62:2141–2150[PubMed]Google ScholarCrossref 9.Voight BF, Peloso GM, Orho-Melander M, et al. Plasma HDL cholesterol and risk of myocardial infarction: a mendelian randomisation study. Lancet 2012;380:572–580[PubMed]Google ScholarCrossref 10.Barter PJ, Caulfield M, Eriksson M, et al.; ILLUMINATE Investigators. Effects of torcetrapib in patients at high risk for coronary events. N Engl J Med 2007;357:2109–2122[PubMed]Google ScholarCrossref 11.Schwartz GG, Olsson AG, Abt M, et al.; dal-OUTCOMES Investigators. Effects of dalcetrapib in patients with a recent acute coronary syndrome. N Engl J Med 2012;367:2089–2099[PubMed]Google ScholarCrossref 12.Konstantinova SV, Tell GS, Vollset SE, Nygård O, Bleie Ø, Ueland PM. Divergent associations of plasma choline and betaine with components of metabolic syndrome in middle age and elderly men and women. J Nutr 2008;138:914–920[PubMed]Google ScholarCrossref 13.Rhee EP, Ho JE, Chen MH, et al. A genome-wide association study of the human metabolome in a community-based cohort. Cell Metab 2013;18:130–143[PubMed]Google ScholarCrossref 14.Illig T, Gieger C, Zhai G, et al. A genome-wide perspective of genetic variation in human metabolism. Nat Genet 2010;42:137–141[PubMed]Google ScholarCrossref 15.Cheng S, Rhee EP, Larson MG, et al. Metabolite profiling identifies pathways associated with metabolic risk in humans. Circulation 2012;125:2222–2231[PubMed]Google ScholarCrossref 16.Magnusson M, Lewis GD, Ericson U, et al. A diabetes-predictive amino acid score and future cardiovascular disease. Eur Heart J 2013;34:1982–1989[PubMed]Google ScholarCrossref 17.D’Agostino RB Sr, Grundy S, Sullivan LM, Wilson P; CHD Risk Prediction Group. Validation of the Framingham coronary heart disease prediction scores: results of a multiple ethnic groups investigation. JAMA 2001;286:180–187[PubMed]Google ScholarCrossref 18.Berglund G, Elmstähl S, Janzon L, Larsson SA. The Malmo Diet and Cancer Study. Design and feasibility. J Intern Med 1993;233:45–51[PubMed]Google ScholarCrossref 19.Manjer J, Carlsson S, Elmstähl S, et al. The Malmö Diet and Cancer Study: representativity, cancer incidence and mortality in participants and non-participants. Eur J Cancer Prev 2001;10:489–49920.Persson M, Hedblad B, Nelson JJ, Berglund G. Elevated Lp-PLA2 levels add prognostic information to the metabolic syndrome on incidence of cardiovascular events among middle-aged nondiabetic subjects. Arterioscler Thromb Vasc Biol 2007;27:1411–1416[PubMed]Google ScholarCrossref 21.Rosvall M, Janzon L, Berglund G, Engström G, Hedblad B. Incidence of stroke is related to carotid IMT even in the absence of plaque. Atherosclerosis 2005;179:325–331[PubMed]Google ScholarCrossref 22.Berglund G, Nilsson P, Eriksson KF, et al. Long-term outcome of the Malmö Preventive Project: mortality and cardiovascular morbidity. J Intern Med 2000;247:19–29[PubMed]Google ScholarCrossref 23.Bonora E, Targher G, Alberiche M, et al. Homeostasis model assessment closely mirrors the glucose clamp technique in the assessment of insulin sensitivity: studies in subjects with various degrees of glucose tolerance and insulin sensitivity. Diabetes Care 2000;23:57–63[PubMed]Google ScholarCrossref 24.Enhörning S, Wang TJ, Nilsson PM, et al. Plasma copeptin and the risk of diabetes mellitus. Circulation 2010;121:2102–2108[PubMed]Google ScholarCrossref 25.Cederholm J, Eeg-Olofsson K, Eliasson B, Zethelius B, Nilsson PM, Gudbjörnsdottir S; Swedish National Diabetes Register. Risk prediction of cardiovascular disease in type 2 diabetes: a risk equation from the Swedish National Diabetes Register. Diabetes Care 2008;31:2038–2043[PubMed]Google ScholarCrossref 26.Lindholm E, Agardh E, Tuomi T, Groop L, Agardh CD. Classifying diabetes according to the new WHO clinical stages. Eur J Epidemiol 2001;17:983–989[PubMed]Google ScholarCrossref 27.Ludvigsson JF, Andersson E, Ekbom A, et al. External review and validation of the Swedish national inpatient register. BMC Public Health 2011;11:450[PubMed]Google ScholarCrossref 28.Centre for Epidemiology, National Board of Health and Welfare. A Finger on the Pulse: Monitoring Public Health and Social Conditions in Sweden 1992-2002. Stockholm, National Board of Health and Welfare, 200329.Wettermark B, Hammar N, Fored CM, et al. The new Swedish Prescribed Drug Register—opportunities for pharmacoepidemiological research and experience from the first six months. Pharmacoepidemiol Drug Saf 2007;16:726–735[PubMed]Google ScholarCrossref 30.Enhörning S, Bankir L, Bouby N, et al. Copeptin, a marker of vasopressin, in abdominal obesity, diabetes and microalbuminuria: the prospective Malmö Diet and Cancer Study cardiovascular cohort. Int J Obes (Lond) 2013;37:598–603[PubMed]Google ScholarCrossref 31.Lyssenko V, Jonsson A, Almgren P, et al. Clinical risk factors, DNA variants, and the development of type 2 diabetes. N Engl J Med 2008;359:2220–2232[PubMed]Google ScholarCrossref 32.Binzak BA, Wevers RA, Moolenaar SH, et al. Cloning of dimethylglycine dehydrogenase and a new human inborn error of metabolism, dimethylglycine dehydrogenase deficiency. Am J Hum Genet 2001;68:839–847[PubMed]Google ScholarCrossref 33.Moolenaar SH, Poggi-Bach J, Engelke UF, et al. Defect in dimethylglycine dehydrogenase, a new inborn error of metabolism: NMR spectroscopy study. Clin Chem 1999;45:459–464[PubMed]Google ScholarCrossref 34.Ho JE, Larson MG, Vasan RS, et al. Metabolite profiles during oral glucose challenge. Diabetes 2013;62:2689–2698[PubMed]Google ScholarCrossref 35.Sheikh K, Camejo G, Lanne B, Halvarsson T, Landergren MR, Oakes ND. Beyond lipids, pharmacological PPARalpha activation has important effects on amino acid metabolism as studied in the rat. Am J Physiol Endocrinol Metab 2007;292:E1157–E1165[PubMed]Google ScholarCrossref 36.Wang W, Wu Z, Dai Z, Yang Y, Wang J, Wu G. Glycine metabolism in animals and humans: implications for nutrition and health. Amino Acids 2013;45:463–477[PubMed]Google ScholarCrossref © 2015 by the American Diabetes Association. Readers may use this article as long as the work is properly cited, the use is educational and not for profit, and the work is not altered.
Supplementary data
Supplementary Table– pdf file
Leave a Reply